Computer-Aided Drug Design Methods PMC
Table Of Content
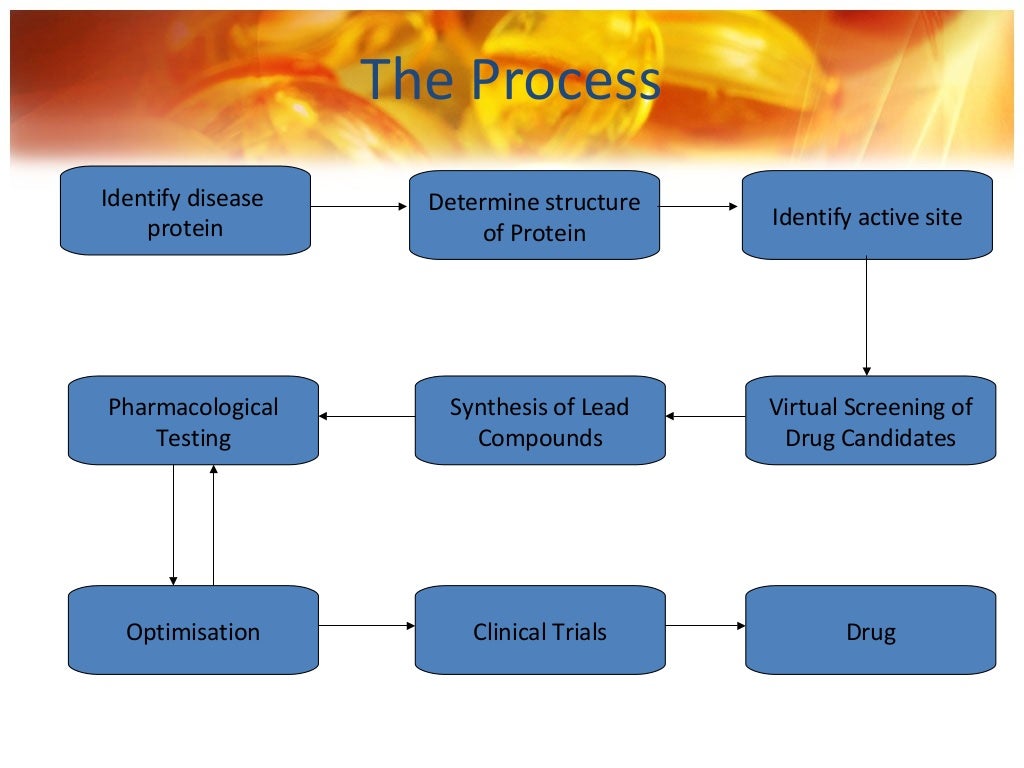
In fact, an average of only 40% of lead/drug candidates passes the different phases of clinical trials and obtains approval for clinical use. Computer-Aided Drug Design (CADD) emerged as an efficient means of identifying potential lead compounds and for aiding the developments of possible drugs for a wide range of diseases [8, 9]. Today, a number of computational approaches are being used to identify potential lead molecules from huge compound libraries. A pharmacophore model is defined as spatially distributed chemical features that are essential for specific ligand-target binding. It represents a simplification of the detailed energetic information used by docking methods and so its computational requirements are much lower. While multiple methods can be used to generate pharmacophores (84), we will present a method based on information from SILCS as described in section 3.2.
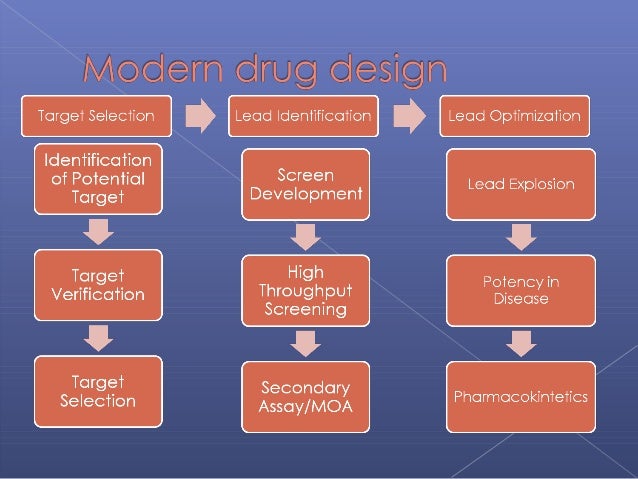
AIIMS, New Delhi 2 day training in Drug Discovery Technology
Other parameters like kinetics include thermodynamics, pharmacodynamics along with higher improvement in ADMET properties have also to be considered. Initially applied to discover new chemotypes for cannabinoid receptor CB2 antagonists, V-SYNTHES has shown a hit rate of 23% for submicromolar ligands, which exceeded the hit rate of standard VLS by fivefold, while taking about 100 times less computational resources26. A similar hit rate was found for the ROCK1 kinase screening in the same study, with one hit in the low nanomolar range26. V-SYNTHES is being applied to other therapeutically relevant targets with well-defined pocket structures.
2. Identification of the Ligand Binding Site
Several cavity prediction tools, such as Castro, Q-site Finder, and COACH, can provide the probable location of the binding site in a protein. The evolutionary-based approach takes the information on all related proteins and shows nearly the same binding site. Geometry-based approaches are based on the features, such as shape, hydrophobic surface, and charged surface residues, whereas an energy-based approach uses a probe to identify the favorable binding regions on a protein.
Genetics of human brain development
Here we present an updated protocol based on the use of oscillating μex Grand Canonical Monte Carlo/MD (GCMC/MD) simulations for SILCS (69). The GCMC/MD approach allows for the application of the SILCS method to target systems with deep or occluded pockets such as nuclear receptors and GPCRs (70). In the era of AI-based face recognition, ChatGPT and AlphaFold68, there is enormous interest in applications of data-driven DL approaches across drug discovery, from target identification to lead optimization to translational medicine (as reviewed in refs. 69,70,71). Generative spaces, unlike on-demand spaces, comprise theoretically possible molecules and collectively could comprise all chemical space (see the figure, part c). Such spaces are limited only by theoretical plausibility, estimated as 1023–1060 of drug-like compounds.
Drug screening
With this approach, the many interactions and energies of large systems (e.g., proteins) can easily be calculated with less computational time and resources because electrons are not taken into account. Conversely, interactions of electrons with the nuclei of the compounds should be considered in small systems which require the application of quantum mechanics calculations. Quantum mechanics are based on quantum physics and very accurate electronic properties can be predicted using the appropriate level of theory and basis set. For instance, electron potential maps and frontier molecular orbitals and several parameters related with to them (chemical hardness, softness, ionization potential etc.) can be calculated.
In either case, elaboration of initial fragment hits to full high-affinity ligands is the key bottleneck of fragment-based drug discovery, which requires a major effort involving ‘growing’ the fragment or linking two or more fragments together. This is usually an iterative process involving custom ligand design and synthesis that can take many years134,138. At the same time, structure-based virtual screening can help to computationally elaborate the fragments to match the experimentally identified conformation of the target binding pocket. Most cost-effectively, this approach can be applied when fragment hits are identified from the on-demand space building blocks or their close analogues for easy elaboration in the same on-demand space139.
Dr. Dev Bukhsh Singh is an Assistant Professor at the Department of Biotechnology, Chhatrapati Shahu Ji Maharaj University, Kanpur, India. Holding a Ph.D. in Biotechnology with specialization in Bioinformatics from Gautam Buddha University, he has been actively involved in teaching and research since 2009, and his focus areas include molecular modeling, chemoinformatics, inhibitor/drug design, and in silico evaluation. He has authored numerous research articles and book chapters in the fields of medicinal research, molecular modeling, drug design, and systems biology. He is a member of various national and international academic bodies, and is a reviewer for several international journals.
Computer Aided Drug Design and its Application to the Development of Potential Drugs for Neurodegenerative Disorders
Artificial intelligence (AI) is a type of machine intelligence that relies on the ability of computers to learn from existing data. AI has been used in various computational modeling methods to predict the biological activities and toxicities of drug molecules [97]. Further, AI has wide applications in drug discovery such as prediction of protein folding, protein-protein interaction, virtual screening, QSAR, evaluation of ADMET properties, and de novo drug design [103]. There are two powerful methods widely used in rational drug design which include machine learning (ML) and deep learning (DL) [104]. ML algorithms that have been extensively used in drug discovery include support vector machine (SVM) [105], Random Forest (RF) [106], and Naive Bayesian (NB) [107]. Few examples of the deep learning methods are convolutional neural network (CNN), deep neural network (DNN), recurrent neural network (RNN), autoencoder, and restricted Boltzmann machine (RBN) [4].
QSAR analysis of VEGFR-2 inhibitors based on machine learning, Topomer CoMFA and molecule docking
According to Gibbs free energy equation, the relation between dissociation equilibrium constant, Kd, and the components of free energy was built. Computers have become an important part of every lab and drug discovery or designing processes are no exceptions. In the rest of this chapter which serves as an update to our first edition, recent progresses in our laboratory toward development of novel SILCS based CADD methods will be overviewed.
The bulky compounds which do not fit well within the binding site pocket are rejected during the lead identification procedure. Advancements in science and technology led to the discovery of macromolecules that played a direct or indirect role in a specific disease. In turn, these discoveries guided chemists to design and synthesize novel bioactive compounds increasingly more active than their predecessors. However, getting a druggable compound to market consists of several steps, many challenges and a tremendous amount of work, time and budget. Between 2009 and 2018 the average cost of drug design and discovery was reported to be up to $2.8 billion [1].
Below we present the SILCS-MC docking protocol assuming the user has already run the SILCS simulations and obtained the GFE FragMaps. A Bayesian ML based reweighting protocol is also described for improvement of the predictability of the SILCS method that can be applied when experimental data on a small set of ligands (10 or more) is available (108). One should not forget here that any computational models, however useful or accurate, may never ensure that all of the predictions are correct. In practice, the best virtual screening campaigns result in 10–40% of candidate hits confirmed in experimental validation, whereas the best affinity predictions used in optimization rarely have accuracy better than 1 kcal mol−1 root-mean-square error. Therefore, computational predictions always need experimental validation in robust in vitro and in vivo assays at each step of the pipeline.
Medicinal and toxicological investigation of some common NSAIDs; A computer-aided drug design approach - ScienceDirect.com
Medicinal and toxicological investigation of some common NSAIDs; A computer-aided drug design approach.
Posted: Thu, 29 Jun 2023 19:36:03 GMT [source]
It also explores computational approaches for identifying potential drug targets and for pharmacophore modeling. We should emphasize here that scoring functions in fast-docking algorithms and ML models are primarily designed and trained to effectively separate potential target binders from non-binders, although they are not very accurate in predictions of binding affinities or potencies. Although these methods are much slower, utilization of GPU accelerated calculations28 holds the potential for their broader application in post-processing in virtual screening campaigns to further enrich the hit rates for high-affinity candidates (Fig. 2), as well as in lead optimization stages. Figure 1 illustrates the basic CADD workflow that can be interactively used with experimental techniques to identify novel lead compounds as well as direct iterative ligand optimization (3, 4, 21, 22).
Drug resistance involving modifications of macromolecules in the outer membrane is a common issue that needs to be considered when searching for new antibiotics (122, 123). While bacterial membranes are complex environments with multiple transport and pore proteins, it is of utility to estimate the pure membrane permeability of drug candidates during drug discovery as this may contribute to drug bioavailability. Traditionally, potential of mean force (PMF) free-energy profiles for a compound across membrane lipid bilayers are derived using MD simulations (124). The PMF may then be used together with position-specific diffusion coefficient in the inhomogeneous solubility-diffusion equation (125) to derive effective resistivity, which may be inverted into permeability. Under the SILCS framework, we recently put forward a protocol to calculate permeation related resistant factor of a molecule to cross membranes (126) using LGFE energy profile and is described in the following.
In this chapter an update to our previous chapter is provided with focus on new CADD approaches from our laboratory and other peers that can be employed to facilitate the development of antibiotic therapeutics. As discussed above, physics-based and data-driven approaches have distinct advantages and limitations in predicting ligand potency. Structure-based docking predictions are naturally generalizable to any target with 3D structures and can be more accurate, especially in eliminating false positives as the main challenge of screening. Conversely, data-driven methods may work in lieu of structures and can be faster, especially with GPU acceleration, although they struggle to generalize beyond data-rich classes of targets. Therefore, there are numerous ongoing efforts to combine physics-based and data-driven approaches in some synergistic ways in general95, and in drug discovery specifically96. An alternative approach proposed to building chemical spaces generates hypothetically synthesizable compounds following simple rules of synthetic feasibility and chemical stability.
A recent study, however, found that regardless of neural network architecture, an explicit description of non-covalent intermolecular interactions in the PDBbind complexes does not provide any statistical advantage compared with simpler approximations of only ligand or only receptor that omit the interactions87. Therefore, the good performances of DL models based on PDBbind rely on memorizing similar ligands and receptors, rather than on capturing general information about their binding. One possible explanation for this phenomenon is that the PDBbind database does not have an adequate presentation of ‘negative space’, that is, ligands with suboptimal interaction patterns to enforce the training. Different algorithms are used to calculate the structural properties and conformations of the small molecules or macromolecules in question [7].
Comments
Post a Comment