Computer Aided Design & Drafting CADD Users Manual Caltrans
Table Of Content
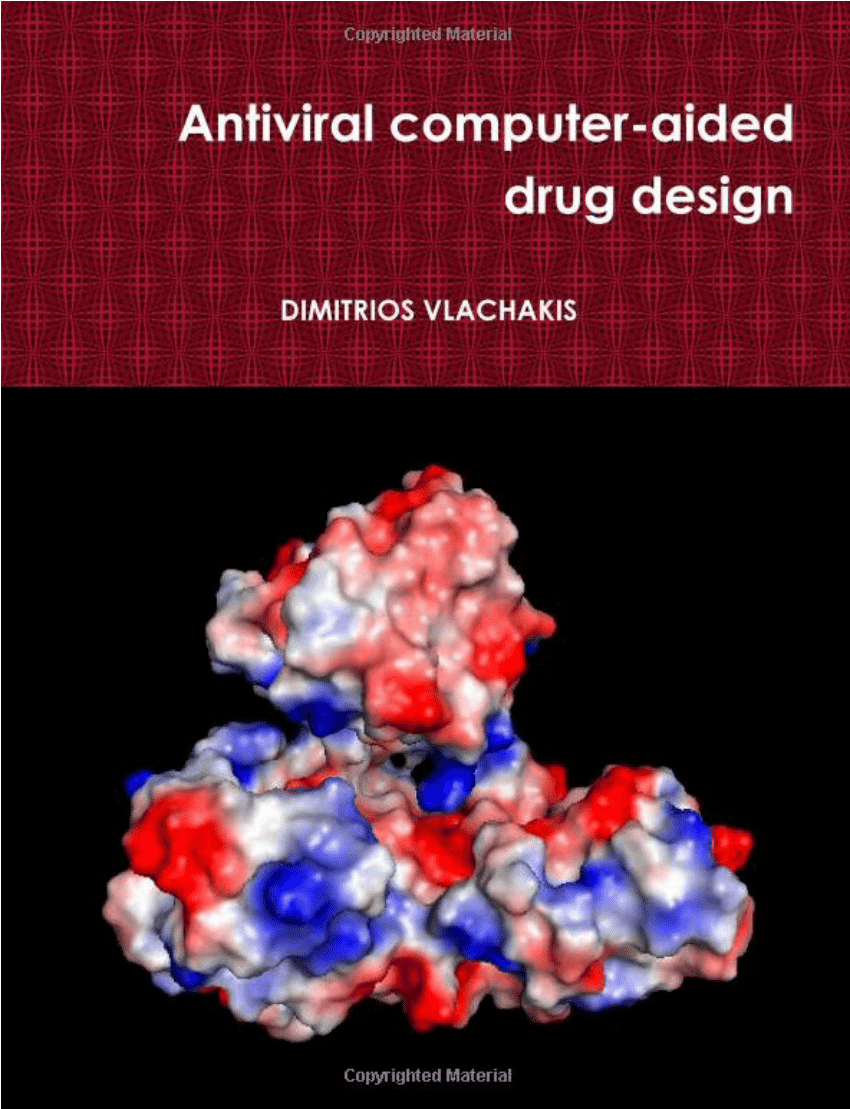
Like SARS and MERS, the genome of SARS-CoV-2 encodes sixteen nonstructural proteins (nsps) such as main protease (Mpro), papain-like protease, RNA-dependent RNA polymerase (RdRp), helicase etc., four structural proteins (envelope, membrane, spike, and nucleocapsid), and other accessory proteins. While the spike glycoprotein is essential for the interaction of the virus with the host cell receptor, the nsps play a major role during the virus life cycle by engaging in the production of subgenomic RNAs [13, 14]. The nonstructural and structural proteins, therefore, offer promising targets for the design and development of antiviral agents against COVID-19 [13]. The lack of effective vaccines or drugs for the treatment of COVID-19 and the high mortality rate necessitates the rapid discovery of novel drugs [15], and computer-aided drug design is believed to be an important tool to achieve the identification of novel therapeutics. There is a possibility of the development of effective lead molecules against COVID-19 by utilizing natural lead molecules obtained through virtual screening and pharmacokinetic prediction [16]. To speed up the discovery of a potential treatment for SARS-CoV-2 infection in humans, repurposing of broad-spectrum antiviral drugs is a promising strategy due to the availability of the pharmacokinetic and pharmacodynamic data of these drugs [17].
5. Molecular Dynamic (MD) Simulation
To overcome this limitation, a new advanced approach was developed and adopted over time which is referred to as computer-aided drug discovery (CADD). Over the course, CADD has accelerated the overall traditional time-consuming process of new drug entity development with the advancement of computational tools and methods. Recently CADD methodologies have become a fundamental and indispensable tool in different junctures of drug discovery and development. Additionally, with the increasing availability and knowledge of biological/bio-macromolecule structures, as well as an exponential increase in computing power, it is now plausible to use these methods effectively without the significant loss of accuracy.
NVIDIA Blackwell Platform Arrives to Power a New Era of Computing - NVIDIA Blog
NVIDIA Blackwell Platform Arrives to Power a New Era of Computing.
Posted: Mon, 18 Mar 2024 07:00:00 GMT [source]
Computational Approaches in Drug Discovery and Design
For example, different protonation states of histidine residues can offer different hydrogen bonding types to potential ligands. Available experimental observations and known complex structures are useful to determine the correct protonation state of protein residue upon ligand binding. Constant pH MD simulation (109) where protonation state of titratable residue can change during the simulation may also be useful. With respect to ligands, many computational tools for prediction of ionization state are available, though common sense by the user is often adequate to deal with the most common ionizable groups such as carboxylates. The knowledge of the binding sites can be extracted from the site-directed mutagenesis study or X-ray crystallographic structures of proteins cocrystallized with substrates or inhibitors [35].
8 Single-step Free Energy Perturbation (SSFEP)
CADD has also paved paths for the screening of selected compounds and synthesis of those entities for better therapeutics. Therefore, CADD is continuously employed with the collective biological and chemical knowledge to rationalize lead optimization, design, and thus can be effectively used in different stages of the discovery and development pipeline. Over the past decades, various CADD techniques such as homology modeling, docking, pharmacophore modeling based virtual screening, conformation generation, ab initio design, toxicity profile, quantitative structure–activity relationship (QSAR), and quantitative free-energy calculation have been greatly improved. The current methods of CADD are routinely utilized in academic and commercial research, as it has been now an emerging field of interest in drug design and developments. This chapter aims to illustrate some crucial CADD techniques also commonly referred to as in silico methods in a diverse arena of drug discovery and focusing some of the modern advancements.
Since then CADD methods have been employed extensively to facilitate the development of novel antibiotics by the computational chemistry community and us for the past five years. This included studies on the mechanism behind antibiotic resistance that may help to guide the design of new antibiotic drugs to overcome such resistance. Al. studied the mechanism of a S222T mutation induced resistance of Deoxy-D-xylulose 5-phosphate reductoisomerase (DXR) to fosmidomycin using molecular dynamics (MD) simulations (5). The MD simulations revealed the structural and energetic basis of the single mutation that induced resistance shedding light on the development of a new antibiotic compounds targeting DXR. Al. recently explored the molecular mechanism of polymyxin E (colistin) resistance in mobile colistin-resistant (mcr-1) bacteria (6). Colistin is the only FDA-approved membrane-active drug to tackle Gram-negative bacteria despite its high toxicity.
3. Docking using SILCS-MC and ML based reweighting for SAR
This synergy is reflected in the latest 3DR Grand Challenge 4 results for ligand IC50 predictions59, in which the top methods that used a combination of both physics-based and ML scoring outperformed those that did not use ML. Going forward, thorough benchmarking of physics-based, ML and hybrid approaches will be a key focus of a new Critical Assessment of Computational Hit-finding Experiments (CACHE), which will assess five specific scenarios relevant to practical hit and lead discovery and optimization97. The modular nature of on-demand virtual libraries supports further growth by the addition of reactions and building blocks.
Overcoming class imbalance in drug discovery problems: Graph neural networks and balancing approaches - ScienceDirect.com
Overcoming class imbalance in drug discovery problems: Graph neural networks and balancing approaches.
Posted: Sat, 11 Nov 2023 03:26:38 GMT [source]
In this regard, the approach has been utilized in proposing drug candidates against coronavirus disease 2019 (COVID-19). The epidemiology, genome composition, pathogenesis, animal models, diagnostics, and vaccine development with references to various computational biology approaches for MERS-CoV infections have been comprehensively reviewed by Skariyachan et al. (2019) [11]. SARS-CoV-2 is a positive-sense single-stranded enveloped RNA virus approximately 30,000 bp in length which utilizes host cellular machinery to execute various pathogenic processes such as viral entry, genomic replication, and protein synthesis [12]. The availability of the three-dimensional structure of the therapeutic target proteins and exploration of the binding site cavity forms the basis of structure-based drug design (SBDD) [18]. This approach is specific and effectively fast in the identification of lead molecules and their optimization which has helped to understand disease at a molecular level [19].
2. Identification of the Ligand Binding Site
This approach may be of use during the fine tuning of ligand affinity or specificity for a target or as required to improve physiochemical and pharmacokinetic properties without significantly altering desirable properties such as affinity. Docking involves posing a compound in the putative binding site on the target in an optimal way defined by a scoring function in combination with a conformational sampling method (78). Various docking programs are available that differ based on the scoring function used to describe the interaction between small molecule and the target and the conformational sampling method used to generate the binding poses of the ligand on the protein. Here we present a docking protocol using the DOCK program (49) to illustrate the typical docking VS workflow. To answer those questions, we focus on structure-function, dynamics, and pharmacology of the GPCR superfamily, probing it with state-of-the-art structural bioinformatics and molecular modeling tools, and validating the predictions with experimental data from public sources and collaborators.
Further optimization by both structure-based and AI-driven computational approaches, which used more than 10 million MADE Enamine building blocks, led to the discovery of preclinical candidates with cell-based IC50 in the approximately 100-nM range, approaching the potency of nirmatrelvir65. The enormous scale, urgency and complexity of this Moonshot effort with more than 2,400 compounds synthesized on demand and measured in more than 10,000 assays are unprecedented and this highlights the challenges of de novo design of non-covalent inhibitors of Mpro. The chemical compound which exhibits biological or pharmacological properties with therapeutic characteristics can be called a lead molecule.
The SILCS-based CADD method was fully described in the first edition of our chapter (4) as well as another chapter previously published in this same book series (20). Then SILCS-Pharm (21, 22) was conducted to extract important binding patterns from FragMaps and turn them into pharmacophore features at the R1 and R2 subsites of CMY-10. Pharmacophore models were then constructed and used to initiate virtual screenings (VS) against over 750,000 commercially available compounds. Top 10,000 hit compounds from the initial pharmacophore screen were selected for SILCS-Monte Carlo (SILCS-MC) sampling for further binding pose refinement and estimation of the binding affinity based on the ligand grid free energy (LGFE) evaluation. Fingerprint based similarity clustering was then conducted to maximize the chemical diversity of the ranked compounds to be selected for bioassay testing. The best hit compound was then subject to a similarity search for chemically similar analogs and more inhibitory compounds were identified.
This molecule is structurally divergent from conventional antibiotics and was confirmed to display bactericidal activity against a wide phylogenetic spectrum of pathogens, demonstrating how ML can guide the antibiotics discovery. In silico screening by docking molecules of the virtual library into a receptor structure and predicting its ‘binding score’ is a well-established approach to hit and lead discovery and had a key role in recent drug discovery success stories11,17,51. The docking procedure itself can use molecular mechanics, often in internal coordinate representation, for rapid conformational sampling of fully flexible ligands52,53, using empirical 3D shape-matching approaches54,55, or combining them in a hybrid docking funnel56,57. Special attention is devoted to ligand scoring functions, which are designed to reliably remove non-binders to minimize false-positive predictions, which is especially relevant with the growth of library size. Blind assessments of the performance of structure-based algorithms have been routinely performed as a D3R Grand Challenge community effort58,59, showing continuous improvements in ligand pose and binding energy predictions for the best algorithms. Several approaches have been developed recently to push the library size limits in HTS, including combinatorial chemistry and large-scale pooling of the compounds for parallel assays.
Building computationally driven pipelines will also help to reveal weak or missing links, in which new approaches and additional data may be needed to generate improved models, thus helping to fill the remaining computational gaps in the DDD pipeline. During the recent era of drug design, many therapeutically potent and well accepted drug targets with unknown active site geometries have been identified. The elucidation of common pharmacophore features is conducted by aligning conformational models and active compounds three dimensionally. A superimposition algorithm assembles training set compounds (3D structure) in the same position/arrangement of their respective chemical properties/features. Pharmacophoric features are positioned such that all/maximum compounds share a common chemical functionality. To refine a shared pharmacophore feature, information regarding inactive compounds can be included in the model generation process.
The primary objective of CADD is to screen, optimize and evaluate the activity of the compound against the target. It forms the multi-disciplinary approach for both academic and major pharmaceutical companies for better efficacy with no/fewer side effects. To speed up virtual screening of ultra-large chemical libraries, several groups have suggested hybrid iterative approaches, in which results of structure-based docking of a sparse library subset are used to train ML models, which are then used to filter the whole library to further reduce its size.
Some of the common methods employed in SBDD include structure-based virtual screening (SBVS), molecular docking, and molecular dynamics (MD) simulations. These methods find numerous applications such as assessment of binding energetics, protein-ligand interactions, and conformational changes in the receptor upon binding with a ligand [20]. Being used by many pharmaceutical industries and medicinal chemists, SBDD as a computational technique has greatly helped in the discovery of several drugs available in the market. The basic steps involved in SBDD consist of the preparation of target structure, identification of the ligand binding site, compound library preparation, molecular docking and scoring functions, molecular dynamic simulation, and binding free energy calculation (Figure 1). The recent outbreak of the deadly coronavirus disease 19 (COVID-19) pandemic poses serious health concerns around the world.
Comments
Post a Comment